A Neuro-AI Framework for Visual Neuroscience
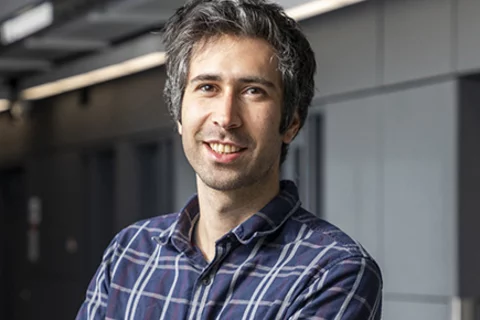
I aim to develop computational models that mimic the transformation between visual stimuli and the activations of retinal, thalamic, and cortical neurons. These models can be used as “digital twins” of the biological visual system, enabling us to predict how different manipulations — e.g., the ablation of a given adaptational mechanism or cell type — affect visual perception. While artificial neural network (ANN) models from the field of AI are the leading type of model in this space, conventional ANN models suffer from two key limitations: 1) they do not capture the retina’s adaptation to changing stimulus conditions, and thus they perform poorly in highly variable settings like those that are encountered in natural vision; and 2) it can be difficult to use them to understand mechanisms underlying visual perception because of the challenge in relating components of abstract ANN models to the biophysical components of the real eye and brain. To address these challenges, my lab has begun to develop hybrid neuro-AI models that incorporate biophysically-detailed models of key retinal neuron classes into fully-trainable AI architectures. In this talk, I will show how these hybrid neuro-AI models enable us to overcome the key challenges in using AI to build digital twins of the visual system.
Presented by: Joel Zylberberg, PhD
Associate Professor, Physics and Astronomy, York University Toronto Canada
Faculty Host: Alapakkam P. Sampath
Faculty Coordinator: Roxana A. Radu, MD
Meeting ID: 986 5616 9804
Password: 416532